What is optical character recognition?
Optical Character Recognition (OCR) is a technology that recognizes printed or handwritten characters from digital images or scanned documents and converts them into machine-readable text.
This technology has revolutionized document processing, enabling the extraction of information from paper-based documents and converting it into editable and searchable digital formats.
OCR systems use advanced algorithms to analyze the shape, size, and location of characters in an image, matching them to a database of known characters. The result is the transformation of visual data into readable text.
Advancements in OCR technology driven, by machine learning and AI, have significantly improved its accuracy.
OCR is now widely used in applications such as document scanning, data entry automation, and text-to-speech technology for people with visual impairments.
Optical character recognition at Pieces
At Pieces, we’ve worked on fine-tuning OCR technology specifically for code.
We use Tesseract as the primary OCR engine, which performs layout analysis before using LSTM (Long Short-Term Memory) trained on text-image pairs to predict the characters.
Tesseract is one of the best free OCR tools, supporting over 100 languages, some of our users used OCR+Pieces to build their own tool.
However, its out-of-the-box capabilities are not ideal for code, which is why we enhanced it with specific pre- and post-processing steps.
Standardized inputs through image pre-processing
To best support software engineers when they want to transcribe code from images, we fine-tuned our pre-processing pipeline to screenshots of code in IDEs, terminals, and online resources like YouTube videos and blog posts.
Since programming environments can be in light or dark mode, both modes should yield good results.
Additionally, we wanted to support images with gradients or noisy backgrounds, as might be found in YouTube programming tutorials or retro websites, as well as images with low resolution, for example, from being compressed from uploading or sending a screenshot.

Since Tesseract's character recognition in image processing works best on binarized, light-mode images, we needed to invert dark-mode images in pre-processing.

To determine which images are in dark mode, our engine first median-blurs the image to remove outliers and then calculates the average pixel brightness.
If it is lower than a specific threshold, it is determined to be dark and thus inverted.
To handle gradient and noisy backgrounds, we use a dilation-based approach.
We generate a copy of the image and apply a dilation kernel and a median blur on it.
We then subtract this blurred copy from the original image to remove dark areas without disturbing the text in the image.
For low-resolution images, we upsample the image depending on the input size using bicubic upsampling.
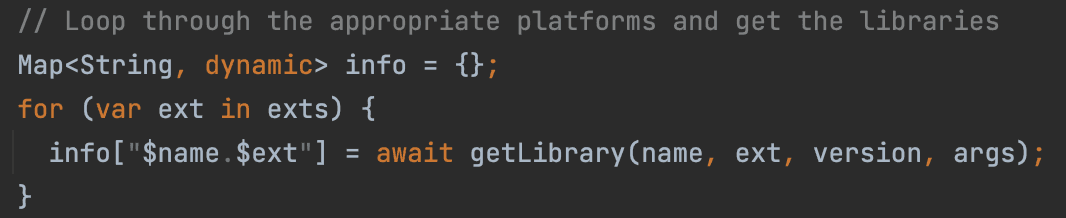

The code requires layout formatting
On the text prediction of Tesseract, we perform an OCR layout analysis and infer the indentation of the produced code.
Tesseract, by default, does not indent any output, which can not only make code less readable but even change its meaning in languages such as Python.
To add indentation, we use the bounding boxes that Tesseract returns for every line of code.
Using the width of the box and the number of characters found in it, we calculate the average width of a character in that line.
We then use the starting coordinates of the box to calculate by how many spaces it is indented compared to the other code lines.

After that, we use a simple heuristic to push the indentations into even numbers of spaces.

Evaluating our pipeline
To evaluate our modifications to the OCR pipeline, we use multiple sets of hand-crafted and generated datasets of image-text pairs.
By running OCR on each image, we then calculate the Levenshtein distance between the predicted text and the ground truth.
We treat each modification as a research hypothesis and then use experiments to validate it.
For upsampling small images, for example, our research hypothesis was that super-resolution models like SRCNN (Super-Resolution Convolutional Neural Network) would boost OCR performance more than standard upsampling methods like nearest-neighbor interpolation or bicubic interpolation.
To test this hypothesis, we ran the OCR pipeline multiple times on the same datasets, each time using a different upsampling method.
While we found that nearest-neighbor upsampled images yield worse results, we did not find a significant difference between super-resolution-based upsampling and bicubic upsampling for our pipeline.
Given that super-resolution models need more storage space and have a higher latency than bicubic upsampling, we decided to go with bicubic upsampling for our pipeline.

Overall, getting OCR code right is a challenging objective, since it has to capture highly structured syntax and formatting while allowing for unstructured variable names and code comments.
We’re happy to provide one of the first OCR models fine-tuned to code and are continuing to improve the model to make it faster and more accurate, so you can get usable code from your screenshots and continue coding.
To test our model on your code screenshots, download the Pieces desktop app.
If you’re a developer interested in our APIs, email us at [email protected]
We’ve integrating with Github, Cursor and recently implemented MCP.
If you liked this article, you might want to read these ones written by me and some of my peers: